Unstructured Data Guide
Table of contents.
Navigate guide
You may have seen this stat before, but it bears repeating: Approximately 80% of all data is unstructured. Analysts predict that by 2025, there will be 163 zettabytes of data in the world and almost all of it will not be contained in the neat rows and columns of a database.
Unstructured data is everywhere, it’s exploding in volume, and it’s messy. But it’s also a treasure trove for those who can tap into it. Let’s learn more about what exactly unstructured data is, why it matters, and how organizations can make the most of it.
What is unstructured data?
Unstructured data usually comes in the form of text in documents, publications, and digital communication. It often provides important context that, when added to insights gathered from structured data, can sharpen decision-making.
The additional intel provided by unstructured data paints a more complete picture. For example, an enterprise that can capture customer behavior and feedback across all its channels has a much better understanding of what that customer thinks. Multiple calls to customer service representatives after a large purchase could indicate that a customer has growing dissatisfaction with a new product, and that the relationship is in jeopardy. Similarly, extensive positive social media chatter about a new offering in different regions could indicate that it’s time to accelerate production and make that product more available globally.
Structured vs. unstructured data
Structured data comprise numbers or text that can fit into the predefined fields of a relational database management system (RDBMS) such as Oracle or Microsoft SQL Server. This data classification typically resides in the rows and columns of a database. Examples include names and addresses, demographic statistics, smartphone locations, and so forth.
Analyzing structured data is especially useful for showing important relationships. For example, ‘existing customers who have ‘purchased product X’ but have not ‘purchased product Y’. While it is the easiest kind of data to search and analyze, structured data represents only about 20% of all data.
As mentioned previously, the much larger proportion of data is unstructured data like word documents, emails, and other text formats. Locating all of the available unstructured data, and then extracting relevant information from it is far more difficult. Since it can’t be organized into a row-column database, tools such as regression analysis and pivot tables won’t work. As a result, organizations often make the mistake of ignoring their unstructured data.
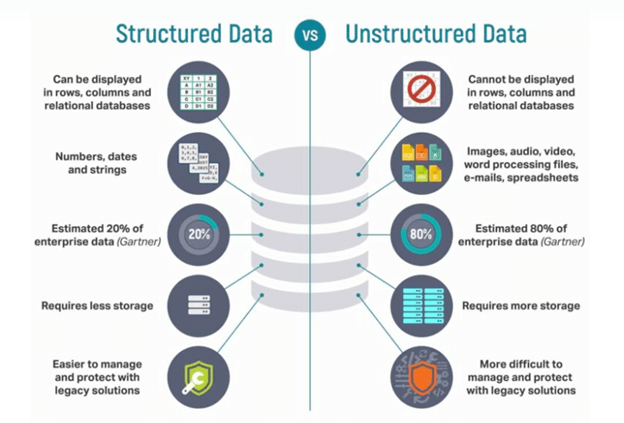
Read the article on Structured vs Unstructured Data: The Ultimate Guide.
Examples of structured and unstructured data
There are many examples of structured data. Here are just a few:
- Lead and sales data in customer relationship management (CRM) systems
- Procurement, inventory, and other data in enterprise resource planning (ERP) systems
- Planning, material sourcing, manufacturing and logistics, and other information in supply chain management (SCM) systems
- Location data from smartphones
- Star ratings by customers
- Demographic information
Common examples of unstructured data include (but are not limited to):
- Emails, which provide a treasure trove of insight into why and how decisions are made
- Mobile and communications data, including texts, chats, and instant messages
- Social media sentiment, including likes, emojis, and comments, which can provide insights into customer needs and behavior
- Digital media, including pictures, audio recordings, and videos
- Text files, such as Word documents, presentations, call center transcripts, surveys, and log files
- Web pages
Why is unstructured data important?
Why should you care about unstructured data? First, organizations have more of it than any other kind of data. And second, although it’s more difficult to collect, process, search, and analyze than its structured counterpart, unstructured data tends to contain a great deal of hidden value.
For example, much of what marketers call “brand sentiment” is buried in unstructured data. You might be able to detect a problem in customer loyalty using structured data sets like sales ledgers. If customers are making fewer repeat orders, that might indicate a brand sentiment problem. Negative brand sentiment would be a lot easier to confirm if an analysis of social media posts revealed that nine of out ten comments contained phrases like “this product is awful.” To see this full picture, you have to have a means of analyzing unstructured data.
Another compelling reason to analyze unstructured data is for the purpose of data classification. Data classification is the practice of identifying and then labeling data according to classifications, such as “intellectual property” or “IP,” “confidential,” “personally identifiable information” or “PII” and the like. Data classification is foundational for data security and compliance. It is nearly impossible, after all, to be effective in protecting data if you don’t know what it is or what it means.
Most serious data security programs make a priority of defending a company’s “crown jewels,” its most valuable and sensitive information. To know what is, and what isn’t, in the crown jewels, it is first necessary to look at all possible data sets and identify which pieces belong in this highly protected classification. Doing this right means examining unstructured data.
For example, your company might put a premium on protecting its patents. That sounds simple enough, but what if information that supports your patent applications is spread out across your entire enterprise? Documents lurking in file drives and cloud volumes could hold rich intellectual property, such as engineering drawings and research reports competitors could steal.
You are vulnerable through your unstructured data. To protect your IP, you need to analyze your unstructured data and find out where all your IP is hidden, and then classify it as IP so it can be properly guarded.
Compliance presents a similar use case. Regulations like the HIPAA law, which protects patient privacy, and the GDPR and CCPA laws that aim to keep consumers’ PII private, require analysis of unstructured data.
For instance, PII data might easily exist inside email messages or PDF documents. If you don’t know it’s there, you can’t guard it against data breaches or unauthorized access. You are then exposed to the risk of significant financial penalties. With the California Consumer Privacy Act (CCPA), for example, a breach of just 400 PII records can lead to a $1 million fine!
Where is my unstructured data?
One of the biggest issues with unstructured data is how to get one’s arms around it. It lives in many places, in many formats. It may be hiding in plain sight, or locked away in a silo. Here are just some of the places where organizations can find their most valuable unstructured data:
Word documents, Excel spreadsheets, PowerPoint presentations, emails, logs, data from Facebook/Twitter/LinkedIn/YouTube/Instagram, website pages, blog posts, text messages, location data, chats, IMs, call transcripts, chatbot conversations, collaboration tools, photos, audio, and video.
In addition to this human-generated unstructured data, many organizations also have access to machine-generated unstructured data. This can include things like satellite imagery, scientific data, surveillance images, and sensor data.
The good news is that big data platforms like Hadoop clusters and NoSQL databases have made it possible to store and manage massive quantities of disparate unstructured data, no matter the source or format. The challenge that remains is analyzing it to turn data into insights.
How do you analyze unstructured data?
Analyzing unstructured data requires the right tools. You have a variety of options, but in general, a modern enterprise search solution will do the job.
An enterprise search solution can conduct data discovery for unstructured data. This means having software “crawlers” go through all of your organization’s unstructured data. The crawlers will parse the contents of Microsoft Office documents, PDFs, email servers, and any other source of unstructured data.
<p”>As data about the documents come back to the enterprise search engine from its crawlers, the solution can build a searchable index of unstructured data. Then, using either built-in features or third-party tools, the enterprise search solution can add data classifications to the unstructured data it has indexed.
In this workflow, it can be helpful to employ a Natural Language Processing (NLP) function that reads the documents like a human being, not a machine. A good NLP solution picks up on nuances within unstructured data that might elude a more traditional, mechanical search application.
For more complete insights, an analytics platform that is compatible with unstructured data may also be required. An analytics platform with this capability usually comprises a connected set of applications, rather than a single solution. It might combine data analytics and visualization with Business Intelligence (BI), NLP, and enterprise search. The analytics platform might also include Artificial Intelligence (AI) functionality that enables it to improve its accuracy and recognize patterns in unstructured data.
What are the challenges of unstructured data?
According to a 2022 study of enterprise IT leaders, more than half of organizations are managing 5PB of data or more, which is up more than 10 points from just one year ago. Eight out of 10 respondents also said that managing unstructured data growth is a top priority.
Unstructured data is growing exponentially year over year. On top of that, with the post-pandemic shift to more remote work, it has been increasingly hard for employees to find the data they need. In fact, a recent Sinequa survey noted that over 60 percent of enterprise employees in the United Kingdom find it more difficult to find data when working remotely.
Why? The data is located in different systems and accessing these systems takes longer at home. Moreover, they find it difficult to ask co-workers for help when not in an office environment.
To avoid these types of inefficiencies, it is extremely important to employ one efficient and powerful data search platform. The search platform should securely access any content from any type of location. For instance, it should be able to conduct a powerful search regardless of whether it is a cloud app like Slack, a PDF, or a spreadsheet. Machine learning capabilities are a must-have as they will significantly help the user experience improve over time as your company evolves.
What value can unstructured data bring to your business?
Quite simply, unstructured data that can be mined, analyzed, and turned into insights can have a direct impact on an organization’s bottom line.
When it comes to business analytics, according to a Qlik/IDC study, three out of fou r companies that invested in data management and analytics increased their revenue, operational efficiency, and profitability by over 15%.
A single, powerful enterprise search engine can extract insightful information from your unstructured data. This allows employees to find the info they need quickly and efficiently. In turn, your employees can focus their energies more fully on profit-making activities – whether they are working in-house or remotely. They, of course, will be able to make better decisions when they have the most relevant, up-to-date information at their fingertips.
Along similar lines, if your employees can access accurate, real-time information, it can lead to timely innovation. For instance, employees will recognize business opportunities and armed with relevant insights, your company can gain a first-mover advantage.
Relevant information also allows your employees to be more responsive to your customers’ needs. Having quick access to data can eliminate supply-chain issues and offer better turnaround times. Customer service employees can provide more timely and accurate information to customers as well. In turn, both the customers and the employees benefit as the customers will appreciate the quick feedback while the employees enjoy increased job satisfaction.
An enterprise-wide search tool also reduces redundancy within an organization. For instance, when using the search tool, your employees will no longer waste time duplicating documents or other assets that already exist within your organization. It also can connect individuals and teams working on similar projects. Further, the enterprise search platform allows your business to streamline processes – saving your organization both time and money.
Companies generate massive amounts of data each day. This data will only continue to increase in the coming weeks, months, and years. While it’s great that more companies recognize the importance of data and consciously save it, this data is only useful if it can be properly analyzed and searched.
Fortunately, there is a powerful way to both find and learn from unstructured data with the use of an enterprise-wide search platform. An enterprise search tool such as Sinequa’s provides companies with valuable insights, saves time and money, and helps companies become more profitable.