3 Ways Manufacturers Can Leverage Insight Engines
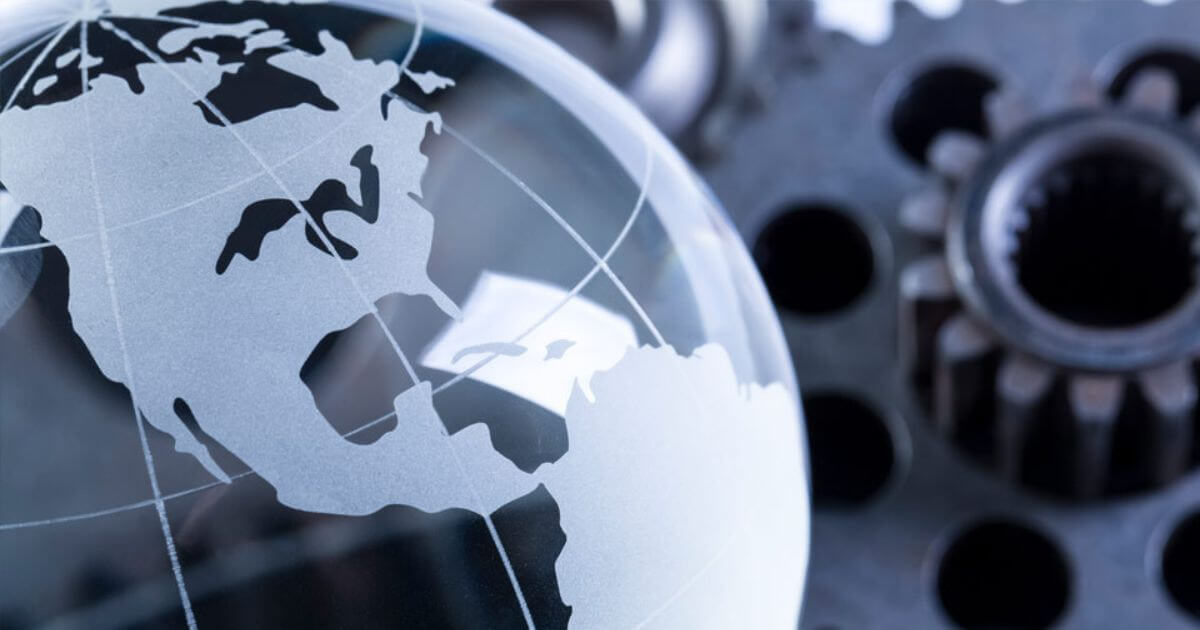
As distributed manufacturing gains adoption by some of the world’s largest companies, products are finding their way into the hands of customers faster than ever before. But in the process, companies are becoming increasingly disconnected and less efficient in new areas. Unlike in traditional manufacturing, where materials are assembled in remote, centralized factories and shipped to customers, distributed manufacturing takes place at multiple, decentralized plants where products are assembled closer to the customer.
The process of leveraging a network of geographically dispersed manufacturing facilities connected over the Internet helps eliminate many of the inefficiencies of traditional manufacturing. However, distributed manufacturing creates new challenges in the form of separate entities, languages and processes.
Teams once gathered under a single roof are now spread across multiple sites, working on projects and programs unique to their local area. These distributed teams are inadvertently scattering knowledge and data across continents and systems, creating information silos that leave workers disconnected.
Content and data have been scattered across a myriad of applications and repositories without any means for end users to surface accurate information with any confidence. In some cases, access to critical institutional knowledge, insights and innovation is restricted to a small few. As a result, disparate teams are spending countless hours making and solving the same problem over and over again, lowering productivity and delaying projects.
A digital solutions manager at a global manufacturing firm once told me, “We had thousands and thousands and thousands of places where our documents were shared, managed by check-in, check-out. In fact, we once had thousands of applications with no search capabilities.”
But once information is accessible from a single place, there are no longer countless teams scavenging for data and insights. With insight engines, manufacturers are bringing the best people together for new projects. Critical parts are easier to locate instead of rebuilding or re-designing them. Even proposals and RFP responses are being developed faster with information found and shared from other RFPs.
These improvements are the result of insight engines that combine cognitive analytics, artificial intelligence and NLP (natural language processing) to helps computers understand, interpret and manipulate human languages, dialects and patterns.
This technology helps manufacturers quickly respond to changing conditions and identify products, parts and components across multiple data sources despite their distance from one another. Here are three ways manufacturers can leverage insight engines to rediscover their synergies and avoid mistakes.
Building Teams Through a Patchwork of Data
Many companies struggle to create the best teams for new projects because expertise is hidden in large organizations with multiple divisions and product groups. Sometimes this task is made more difficult by employees who fail to enter their background and experience in their HR profiles or update them with new skills or certifications.
AI-powered insight engines leverage NLP to surface information from large volumes of data stored in hundreds of millions of files, and thousands of repositories and databases to uncover work histories, experience on different projects, expertise, training and education in order to locate experts. Projects employees have worked on, languages they write in, and locations where they’ve traveled are all easily queried and analyzed to identify experts within the company.
Avoiding Duplication of New Parts
In a distributed environment, it’s difficult to find parts across systems and continents. Sometimes it’s easier to make new ones. That’s because parts are generally indexed by labels, which can be difficult to identify correctly. As a result, the exact same part is recreated, sometimes up to five times, each version with its own label.
This is a complete waste of time and money. And when a part is recreated, there’s a greater chance of a new flaw being introduced, which can be catastrophic in industries like transportation or medical devices.
With insight engines, parts data can be contextualized for engineers to identify duplicate parts and eliminate duplications. For any company with a broad base of engineers, the cost savings from this approach can be enormous.
Increasing the efficiency of proposal development
Companies with multiple departments and divisions often fail to share technical information for sales proposals and RFPs. Consequently, proposals for an opportunity in one part of the world with many of the same requirements as others in different markets or regions must be developed from scratch.
And, if questions arise during the proposal process, it is time-consuming and difficult to get answers from engineers. Acquiring new customers in a complex environment with a highly engineered, configurable project is time-consuming by its nature. The inaccessibility of data makes it worse.
Insight engines surface information in context across emails, databases, and records. With this information in hand, companies can respond faster to sales opportunities and present a common face for the company overall.
In all three of these scenarios, information and insights are securely surfaced from content and data in every application and every repository from every location across globally distributed companies, which enables teams to cultivate, improve quality and take advantage of new opportunities.