Everything You Need to Know about Insight Engines
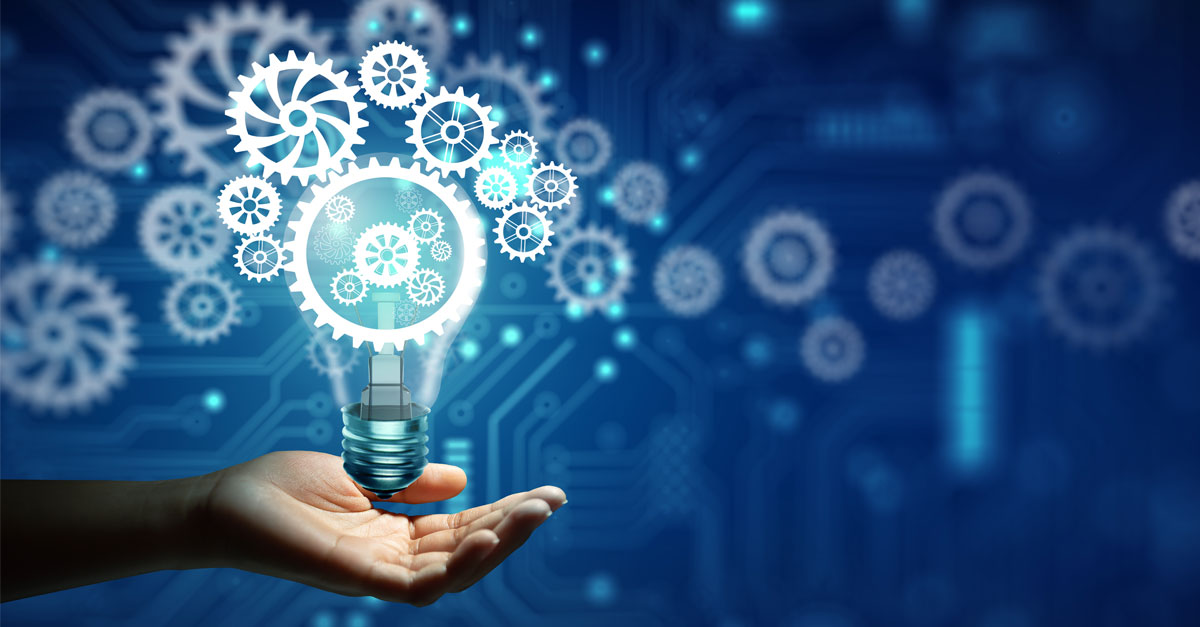
In recent years, the idea of enterprise search has evolved from simple text-based keyword-type queries, giving way to far more sophisticated capabilities. The latest incarnation is known as the “Insight Engine.” The term, coined by the Gartner analyst firm, describes Insight Engines as solutions that combine search indexing across multiple data sets with artificial intelligence (AI), natural language processing (NLP), machine learning, deep learning, and other technologies. Users become able to discover information that’s more relevant and comprehensive than was previously possible.
What is an Insight Engine?
According to Gartner, “Insight engines apply relevancy methods to describe, discover, organize, and analyze data. This allows existing or synthesized information to be delivered proactively or interactively to customers or constituents at timely business moments.” Breaking this down a bit, Gartner says that an insight engine pulls together the information most relevant to search queries from a broad set of sources. These include structured and unstructured data sets.
Insight engines are gaining traction as a new generation of search engines because they do more than earlier search technology to address the core, inherently human dimensions of business. If enterprise search helps employees find information, insight engines speak to why an employee is seeking information—and provide knowledge that will satisfy the reasons for the search in the first place.
Essentially, insight engines work on answering questions that are not being explicitly asked. When a wife asks her husband, “Are you sure you want to wear that tie?” the question is about a lot more than the tie. So, too are most search queries about more than their surface parameters.
A customer calling a support line has a problem. They are not calling to say, “Hi, how’s the weather?” They’re calling for a reason, usually related to the quality of their experience. The faster and more accurately the customer service representative can understand and solve that problem, the better off the business will be.
A better name for this technology might be “implication engines,” as the insight engine is designed to investigate the implication of a search query.
Consider an example. Suppose a wealth advisor’s client asks whether she should own a particular stock. In that case, the implications of this question run the gamut from how well the advisor manages her wealth, how well-informed the advisor will look to the client, and how the advisor’s firm will fare with this client in the long term. There’s more riding on this question than just “buy the stock” or “don’t buy the stock.”
For these reasons, the insight engine should be configured to generate search results that answer more than the simple question and do it quickly. A wealth advisor’s insight engine might instantly provide the advisor with extensive but highly relevant information about the stock in question. This enables the advisor to give a detailed answer to this seemingly simple question, a response that speaks to the question’s more severe implications.
Making this work requires extracting information from massive volumes of complex, diverse data. As Gartner said, insight engines “use connectors to crawl and index content from multiple sources. They index the full range of enterprise content, from unstructured content such as word processor and video files through to structured content, such as spreadsheet files and database records.” Gartner adds, “Various ‘pipelines’ are used to preprocess content according to type, and to derive from it data that can be indexed for query, extraction and use via a range of touchpoints.”
In a customer support setting, an insight engine might be employed to generate an instant 360-degree view of a customer. It could gather data from the customer’s order history, emails, customer relationship management (CRM) systems, PDFs of legal agreements, social media accounts and more—giving customer service representatives a complete idea of the customer’s current relationship with the business.
In this way, the insight engine provides in-depth, contextually relevant information about the customer while making the customer service representative more productive. They do not have to go through documents and screens of information to arrive at an accurate sense of the customer’s situation and needs.
The mechanics of the insight engine involve cognitive systems that use NLP to understand the meaning of unstructured data, e.g., from documents, blogs, engineering reports, market research, and rich-media content like videos. Machine learning algorithms further refine the insights and relevance gathered from these data sets.
3 things to consider before choosing an Insight Engine vendor
What should one keep in mind when selecting an insight engine? Many factors come into play, but three stand out: security, integration and scalability. Security is an essential consideration in any IT purchase, of course, but with an insight engine, the nature of the technology exposes the organization to a serious risk of data breach.
An insight engine’s deep and broad access to data makes it a good target for malicious actors. If a hacker can take over an insight engine, he can see a wide variety of data. Indeed, the insight engine will make the hacker’s job easy by directing him to just about any piece of information he wants to steal.
To mitigate this threat, a secure insight engine will offer several specific countermeasures. It will enable fine-grained access controls. After all, not all users should have access to all of the content discoverable by the insight engine.
Implicit in this countermeasure is some system of data classification process. System owners have to identify and segregate sensitive data, such as intellectual property or personally identifiable information (PII) from end users who do not have permission to see such data. Then, the insight engine should have robust privileged access controls, limiting and monitoring administrative access.
The ability to integrate with data sources is essential for insight engine success. The integration has to be as broad-based as possible, with the engine linking with virtually any type of data that might aid in the mission of generating relevant insights. This goes beyond connecting with databases and document repositories.
The insight engine can access email servers, social media platforms, and other unstructured data sources. And the integration has to be easy to achieve and manage. If connectors are difficult to implement and update, that will impair the functioning of the insight engine.
Scalability matters because insight engines must perform consistently as they tap into ever more massive volumes of data. The insight engine cannot be slowed down by an inability to scale. A good insight engine will provide access to its full range of insights to all end users within milliseconds. This may sound like a high bar, but Google does it for consumer search daily, so it’s definitely achievable in an enterprise setting—with the right platform.
Use cases of Sinequa’s Insight Engine
Sinequa’s insight engine is built on a dynamic, extensible platform to support various use cases. These include internal and external search, along with purpose-built insight apps. Internal search use cases put the insight engine to work on internal data, as exemplified by the 360-degree view of the customer.
In addition to pulling in relevant data points from multiple sources of customer information, the internally focused insight engine can also suggest in-house experts to handle a customer matter. For instance, if the customer has a logistics problem, an expert finder can link the user to the company’s logistics experts to address the customer’s needs.
The stock picking example shows how the external search use case can work for an insight engine. Here, the insight engine is directed to harvest relevant data from industry data sources. The engine’s user interface can be customized to show data related to the stock’s trading volume, the company’s board of directors, recent news stories, regulatory filings, sentiment analysis from social media and more.
The platform can be further utilized to build an application based on the insight engine. In the stock picking example, developers can create a risk profiling widget that visualizes risk data discovered by the insight engine in real time. The end user gets the advantage of seeing all of this relevant data about the stock in real time—without having to wade through reports and documents.
Insight engines are gaining popularity as enterprises see the business advantages of using them. They take enterprise search to the next level, answering implicit questions and making the business more responsive to customer needs and employee inquiries. The right insight engine will be secure, scalable, and easily integrative. With an insight engine that meets these criteria, organizations can use all of their data for business advantage.