Leveraging Generative AI in the Workplace
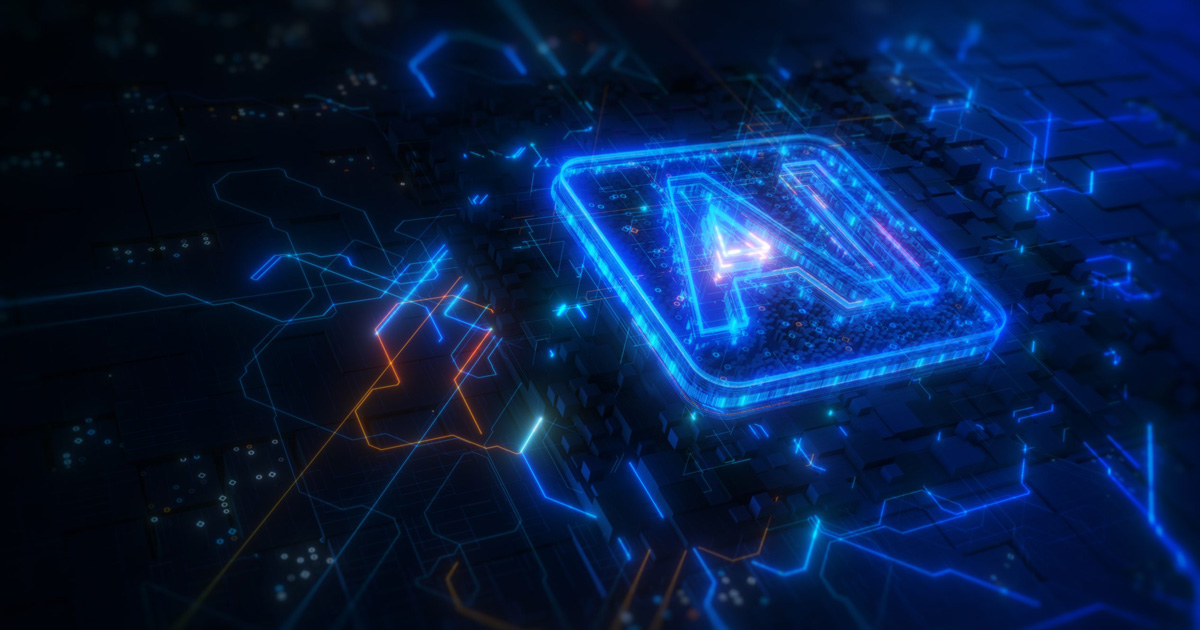
In the swiftly evolving business arena, data stands as the lifeblood empowering organizations. To decipher the deluge of daily data, businesses harness Generative AI—a facet of artificial intelligence (AI)—unleashing actionable insights from intricate and unstructured data sources.
Among the various techniques used in cognitive analytics, GenAI stands out as a powerful tool that can transform raw data into actionable intelligence.
Understanding Generative AI
Generative AI, also known as generative adversarial networks (GANs), is an advanced AI technique that involves two neural networks: a generator and a discriminator. The generator creates new data samples, such as images, text, or even audio, while the discriminator evaluates the authenticity of those samples.
The generator and discriminator engage in a competitive process, where the generator aims to produce data samples that are indistinguishable from real ones, and the discriminator strives to accurately classify real and generated samples. Through this iterative process, both networks improve their abilities, generating highly realistic and original data samples.
Leveraging GenAI for Actionable Business Insights
GenAI holds immense potential in various areas of business intelligence. Let’s explore some key applications where GenAI can be leveraged to extract actionable insights:
1. Synthetic Data Generation
One significant application of GenAI is the creation of synthetic data that mimic real-world scenarios. Synthetic data can be used when real data is scarce, expensive, or subject to privacy restrictions. By using generative AI, businesses can create large volumes of realistic data that closely resemble the characteristics and patterns of their target audience, enabling more accurate analysis and modeling.
2. Data Augmentation
Generative AI can enhance existing datasets by generating additional samples, thereby increasing the diversity and size of the dataset. This augmentation helps improve the robustness and generalization capabilities of machine learning models, leading to more accurate predictions and better decision-making.
3. Anomaly Detection
Generative AI can be employed to detect anomalies or outliers in datasets. Training a generative model on normal data patterns can identify any data points that deviate significantly from the learned distribution as potential anomalies. This enables businesses to proactively detect fraudulent activities, system failures, or any other irregularities that could impact operations.
4. Natural Language Processing (NLP)
Generative AI has shown remarkable capabilities in NLP tasks, such as natural language understanding, text generation, and sentiment analysis. By training on vast amounts of text data, generative models can generate coherent and contextually relevant text, making them invaluable for content creation, customer support chatbots, and personalized marketing campaigns.
5. Image and Video Synthesis
Generative AI has revolutionized image and video synthesis, enabling businesses to create highly realistic visual content. This technology finds applications in product prototyping, virtual environments, and digital marketing campaigns, where generating high-quality visuals is crucial for engaging customers and driving conversions.
6. Predictive Analytics
Generative AI can be used to generate future scenarios based on historical data, enabling businesses to make informed decisions and predict potential outcomes. By generating multiple possible futures, organizations can evaluate various strategies and assess their potential impact, helping them optimize business operations and reduce risks.
Cognitive analytics, combined with the power of GenAI, offers businesses unprecedented opportunities for actionable business insights.