Relevance Feedback is Key to the Information-Driven Economy

As the data-driven age gives way to an information-driven economy where context is critical to surfacing useful insights from data, taking in relevance feedback from users, especially expert users, will play a major role in driving the benefits. This article explains the concept of a relevance feedback model and why you should care.
What is a Relevance Feedback Model?
Assume you ask a person or a system to provide you with information on a certain topic. There may be many facets to this topic, and you may get information from a whole range of different aspects.
If you are working with that person or that system on a permanent basis, you may want to tell them that only certain aspects and hence certain kinds of information are relevant to you – in the hope of getting only the more relevant answers from them the next time you ask. You give the person or system “relevance feedback”.
Now let’s concentrate on a system, a cognitive information retrieval system or a “system of insight”. In that context, a relevance feedback model (RFM) is the capability of the system to take your relevance feedback and “internalize” it in order to tune the results of your future queries to what is most relevant.
The system performs and automates this task by adjusting weights attributed to certain terms and their equivalents (i.e. terms with the same or similar meanings) within the data it processes.
Imagine you asked “what do we have on MRO”, and you got information back on maintenance, repair and operations, but you told the system that you are only interested in anything pertaining to “Mars Reconnaissance Orbiter”. The next time you ask, you will get information only pertaining to the latter and possibly on related topics like Mars landing craft, automated robots for planetary exploration, etc.
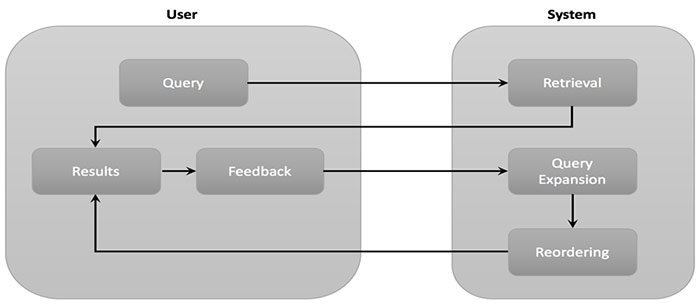
For one person and one query, that seem rather simple. But now imagine, that you have tens of thousands of colleagues and thousands of topics to cover. That is when the RFM benefits from machine learning algorithms, not only to detect the preferences of each person but also of groups of people with similar interests, similarities in documents, etc. to spread the user relevance feedback to other documents, queries and people on an ongoing basis in an automated way.
Why use a Relevance Feedback Model?
A key benefit of a relevance feedback model is to enable users, in particular expert users, to affect relevance appropriate to their environment without the IT department having to implement rules for relevance according to specific user groups. It allows administrators to decide by configuration which specific users within the organization will contribute as well as the exact factor of relevancy improvement.
The relevance feedback model can also go a long way towards improving the human-machine interaction. As the relevance of certain content increases significantly due to relevance feedback, the user experience starts to feel much more “conversational” – i.e offering one to three suggestions as “answers” to a query – than a traditional search interface offering a list of documents in response to a query.
The RFM provides a way to discover from everyone’s experience the information that best answers the question. Take the real-world case of a customer service representative (CSR) seeking an answer to a customer’s product question using the product name or code. In this case, the CSR will obtain a diverse set of documents including parts catalogs, how-to information, product specifications, packaging information, marketing material, etc. All of this information is relevant but only some of it may help the CSR answer the customer’s question.
Thanks to the RFM, the CSR would immediately see information she has already viewed when she searched similar things in the past because the RFM takes into account the user’s “click actions” and applies a tiny relevance boost accordingly.
Perhaps even more powerfully, the RFM will also modify the order of the results by observing (over time) what information other CSRs spend time to discover, even when they dive deeply into the results list for relevant information. Organizations striving to take full advantage of the RFM will configure it so that the experts’ interactions with the system provide bigger boosts for important content and even ban inaccurate information from appearing in results lists.
As you can see from the example above, the RFM provides a collaborative way to modify search result order. It is neither a tagging nor a classification approach, both of which can be done at indexing time (extracting metadata from source, entity extraction with Natural Language Processing or afterwards (classification through ML algorithm like clustering, similarity computation, and so forth). The RFM arguably represents a smarter approach by directly incorporating human decisions when presenting information that will best address a user’s query.
As information-driven organizations strive for ever higher degrees of accuracy for end users seeking knowledge, the ability to leverage relevance feedback from users, especially expert users, automatically at scale becomes increasingly mission-critical for optimal business performance.