Becoming Information-Driven Begins with Pragmatic AI
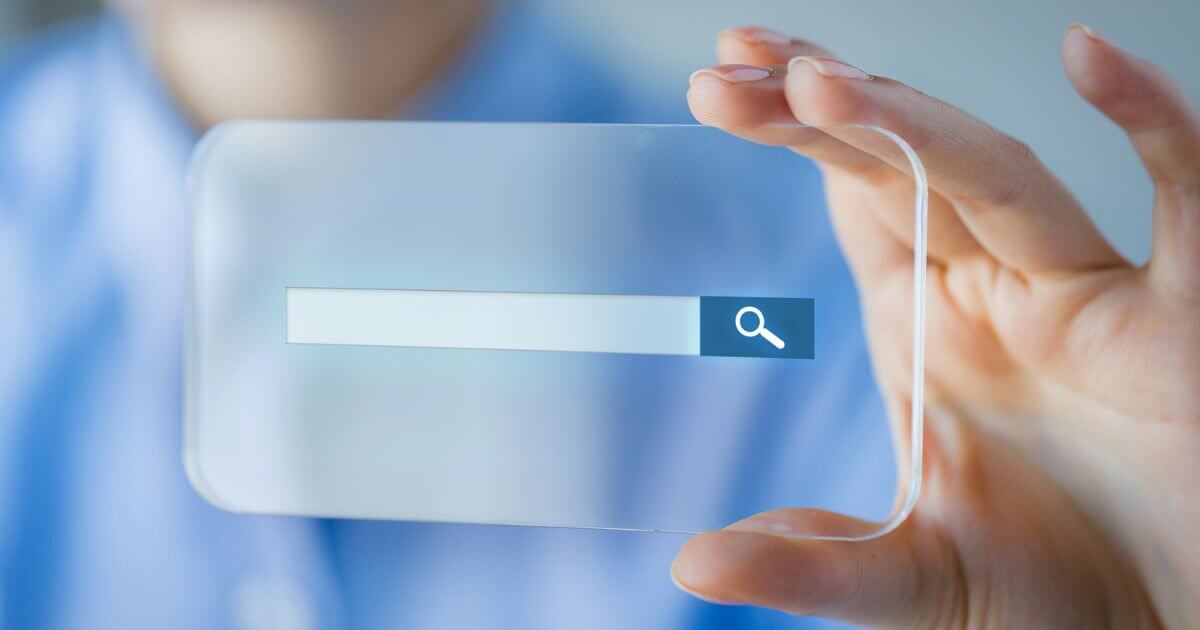
Written by guest blogger, David Schubmehl, IDC Research Director, Cognitive/Artificial
Over the last several years, I’ve spoken to many organizations that have all asked the same question: How can we most effectively make use of all of the research, documents, email, customer records and other information that our employees have collected over the years, especially those that are now retiring?
In the past, organizations had corporate libraries and corporate librarians whose job it was to help collect, organize, and disseminate information to employees and staff when and where they needed it. That department and positions are long gone from most organizations today. Why have they gone? The rate of data and documents (including research papers, contracts, and even emails) has exploded, making this task impossible.
But let’s be honest: even before today’s information explosion, no classification system could ever keep up with the fast pace of change in the economy. No one could have foreseen today’s most important questions, in content categories that did not exist until today. And with the baby boomers retiring at an ever-increasing rate, an urgent question must be asked: How do organizations get the most value from the vast amounts of information and knowledge that they’ve accumulated over decades?
IDC has identified the characteristics of organizations that are able to extract more value out of the information and the data available to them. Leader organizations make use of information access and analysis technologies to facilitate information access, retrieval, location, discovery, and sharing among their employees and other stakeholders. These insight leaders are characterized by:
- Strategic use of information extracted from both content and data assets
- Efficient access to unified and efficient access to information
- Effective query capabilities (including dashboards)
- Effective sharing and reuse of information among employees and other stakeholders
- Access to subject matter experts and to the accumulated expertise of the organization
- Effective leverage of relationships between information from different content and data sources
So how can artificial intelligence (AI) and machine learning affect information access and retrieval? The types of questions that are best answered by AI-enabled information access and retrieval tools are those that require input from many different data sources and often aren’t simple yes/no answers.
In many cases, these types of questions rely on semantic reasoning where AI makes connections across an aggregated corpus of data and uses reasoning strategies to surface insights about entities and relationships. This is often done by building a broad-based searchable information index covering structured, unstructured, and semi-structured data across a range of topics (commonly called a knowledge base) and then using a knowledge graph that supports the AI based reasoning.
AI-enabled search systems facilitate the discovery, use, and informed collaboration during analysis and decision making. These technologies use information curation, machine learning, information retrieval, knowledge graphs, relevancy training, anomaly detection, and numerous other components to help workers answer questions, predict future events, surface unseen relationships and trends, provide recommendations, and take actions to fix issues.
Content analytics, natural language processing, and entity and relationship extraction are key components in dealing with enterprise information. According to IDC’s Global DataSphere model developed in 2018, of the 29 ZB of data creation, 88% is unstructured content that needs the aforementioned technologies to understand and extract the value from it. In addition, most of this content is stored in dozens, if not hundreds of individual silos, so repository connectors and content aggregation capabilities are also highly desired.
AI and machine learning provide actionable insights and can enable intelligent automation and decision making. Key technology and process considerations include:
- Gleaning insights from unstructured data and helping to “connect the dots” between previously unrelated data points
- Presenting actionable information in context to surface insights, inform decisions, and elevate productivity with an easy-to-use application
- Utilizing information handling technologies that can be used in large scale deployments in complex, heterogeneous, and data-sensitive environments
- Enriching content automatically and at scale
- Improving relevancy continuously over time, based on user actions driven by machine learning
- Improving understanding by intelligently analyzing unstructured content
IDC believes that the future for AI-based information access and retrieval systems is very bright, because the use of AI and machine learning coupled with next-generation content analysis technologies enable search systems to empower knowledge workers with the right information at the right time.
The bottom line is this: enabled by machine learning–based automation, there will be a massive change in the way data and content is managed and analyzed to provide advisory services and support or automate decision making across the enterprise. Using information-driven technologies and processes, the scope of knowledge work, advisory services, and decisions that will benefit from automation will expand exponentially based on intelligent AI-driven systems like those that Sinequa is offering.