ChatGPT a Google Killer? Not so fast. Search is More Important than Ever!
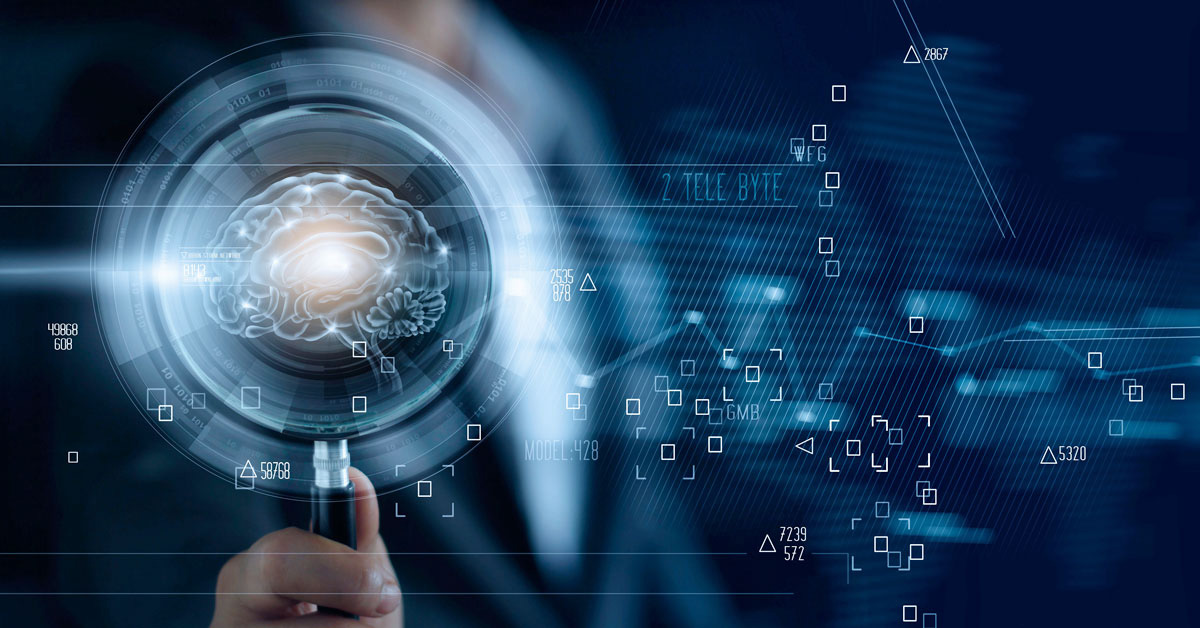
It’s been an exciting few months since OpenAI released ChatGPT. It’s been hailed the next “iPhone moment” – a technological marvel that promises to revolutionize almost everything. We’re only 16 years into the iPhone era, and it’s difficult to think of a part of your life it hasn’t changed…and almost impossible to think of life without it.
At first, ChatGPT was the best thing ever. The most powerful AI we’ve seen. It would take the drudgery from people’s jobs and free students from homework. It would make people more productive and elevate creativity. We’ll never have to search again; it’ll do all the work for us! Watch out Google, because search is dead! Microsoft’s got ChatGPT, and they put it into Bing, and it was so amazing. Everyone was enthralled, search was solved, humanity dumped Google for Bing, and Google quickly went out of business!
Oh no, wait, that’s not what happened.
Instead, everyone is lamenting how bad the new Bing is. It lies while sounding authoritative. It is rude. It makes stuff up. So what’s going on?
ChatGPT and other Generative Large Language Models have Limitations
The basis of all this technology that everyone is so hyped about is a Large Language Model (LLM). It’s a model that understands language patterns – not language itself, but language patterns. But it’s so good at these patterns that it can write text as well as you can. Probably better. It’s called generative because it generates new and unique text every time. And it works well for a whole bunch of things. But alas, not everything.
LLMs and Search
One of the things that a generative LLM is not good at is search. That’s because search is about retrieval – find me information on a topic. A generative LLM doesn’t find anything. Instead, it takes what it’s been trained on and creates (generates) a response based on that training.
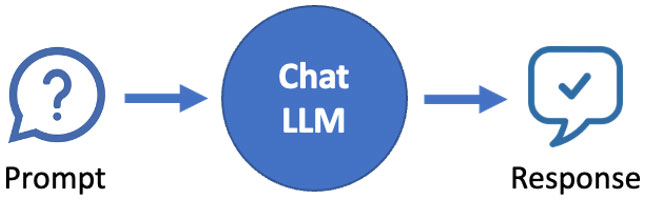
A generative LLM (like a chat LLM) can respond to prompts based on what it was trained on.
Sometimes it feels like search because it is surfacing some of the material it’s been trained on, but unlike search, it’s not the content itself but an imperfect reflection of that content (at its most basic, it’s a mishmash of words created based on probabilities). But if generative LLMs are bad at search, why are Microsoft and Google racing to incorporate them into their search offerings?
Search Powers the Generative LLM
There are many reasons, but the primary reason is that generative LLMs bring new capabilities that go beyond search and will attract customers, which means more eyeballs and more ad revenue. When we go to their new “search” pages we can still search, but we’ll also be able to have it write an email or essay or poem (Bing’s “compose” mode), have a conversation (Bing’s “chat” mode), compile a list, or stylize something we wrote. Those are the primary capabilities of generative LLMs, but don’t have anything to do with search.
But when we do search, a generative LLM can be used to enhance the search results by taking the results and synthesizing them into an easy-to-read summary. Instead of poring over a list of results and snippets and links, we can read a plain-language explanation of the results.
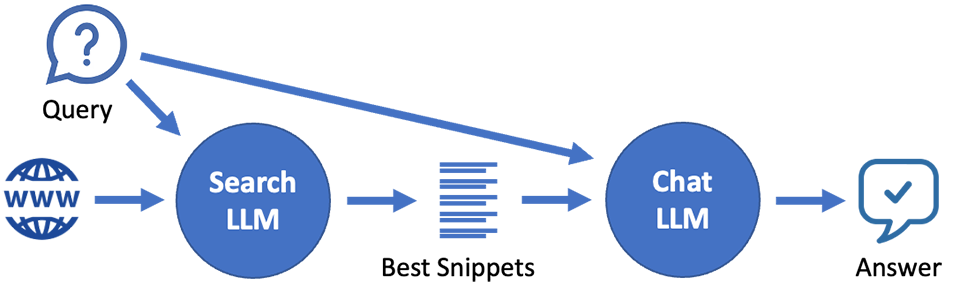
The real power of applying generative LLMs to search is not for search itself but for convenience: to summarize the results into a concise, easy-to-read format.
With this approach, the answer comes NOT from the information that trained the LLM. Instead, it comes from the results of the real-time search. The generative LLM doesn’t answer the query, it summarizes multiple answers into one. This is immensely better than using the model itself because asking the LLM to only use the search results in its response means the answer is:
- current (vs. the LLM, which has no knowledge of anything after the date it was trained)
- more focused and higher quality (vs. the LLM, which is generic and unfocused)
- traceable (parts of the summary can be linked back to the short list of search results, vs. the LLM, which is a black box)
- much more accurate (it has very little “stray” information that could cause a hallucination, vs. the LLM, which draws from unfocused content and is more likely to produce a wrong response)
Search Becomes More Important, not Less
Incorporating generative LLMs makes search even more critical because the summary produced by the LLM is wholly based on the results of the search. Better results means a better summary, and garbage in means garbage out! With a list of ten links, it’s easy for a human to see that result #4 is irrelevant for what they’re after. But the LLM can’t, and will use all ten results to create its response which could easily include that unrelated (or incorrect!) information.
Today’s LLMs are no match for humans’ ability to discriminate information in this way. Until they do, the quality of the search results will dictate the quality of any summary created by an LLM. As people transition from scanning a list of results to expecting a convenient summary, the quality of the search engine is everything. It’s also the only way to fact-check anything that the LLM produces! The links provided in the summary are links to search results; if those links aren’t sufficient, the way to get more information is…to search for it!
What About the Enterprise?
So far, this has been about using generative LLMs like ChatGPT for internet search, something we’re all familiar with. But the same principles apply to search within an organization – enterprise search. Sinequa has always been focused on retrieving the most accurate and relevant results from all content, regardless of source, format, or language. That ability is more important than ever, to take advantage of the exciting capabilities of generative LLMs so that they can produce something that is current, focused, traceable, and accurate.
Using search in this way to feed a generative model adds convenience and shortens the information-gathering process with a significantly reduced risk of hallucinations. We can already do this today by providing search results to a generative LLM (via an API like those available for GPT-3 or GPT-3.5). At the same time, we continue researching new ways to leverage generative AI and LLMs in our products.
Where This is Going
We’re still at the very early stage of understanding what generative LLMs like ChatGPT and Bard will mean for how we interact with information, how we do our jobs, and even how we live and create. While (at this stage) they are not a replacement for search, they can make search more convenient and efficient. This is exactly what Microsoft (Bing) and Google (Bard) are promising, and Sinequa is doing the same for enterprise search. As these tools evolve and improve, we’ll see new applications that we can’t even imagine. A few years from now, much like smartphones, we’ll wonder how we ever got along without them.